Intel helps researchers detect breast cancer
- June 13, 2023
- Steve Rogerson
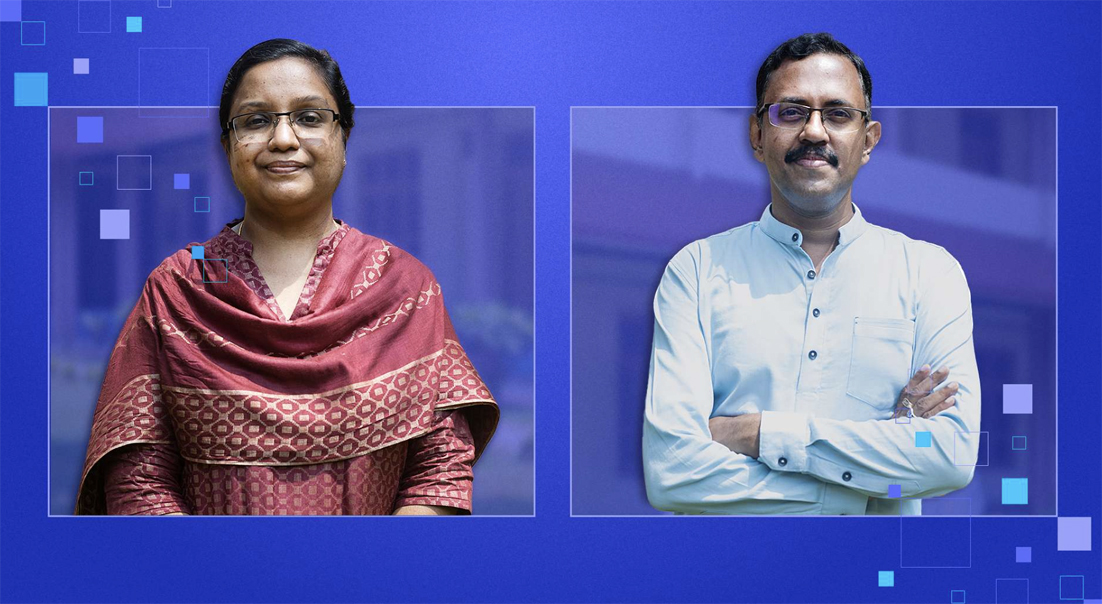
An Intel-backed AI model is helping researchers detect breast cancer with high accuracy.
Researchers Madhu Nair and Asha Das were close to a breakthrough that would use artificial intelligence (AI) models to detect breast cancer cells in scanned images taken from patients’ tissue samples. But a huge problem stood in the path of these two India-based researchers.
They struggled for months to teach their AI model to recognise cancer cells accurately. Das and her team would often spend weeks reviewing stacks of high-resolution mega-pixeled images, marking cancerous areas image by image.
The team needed a method that could scan these images accurately and quickly without human supervision.
In 2022, Das and Nair, researchers from the Artificial Intelligence & Computer Vision Lab at Cochin University of Science & Technology in India, partnered with Intel to implement a method using Xeon scalable processors and the Intel Optimization for TensorFlow, a software suite that leans heavily on the acceleration features in Intel CPUs.
The researchers have created CPU-based technology that can detect the early signs of breast cancer on medical images.
Today, spotting early signs of breast cancer relies heavily on the expertise of radiologists and doctors who manually scan histopathological results. But relying solely on the human eye has its downsides – about a fifth of breast cancer signs are missed, according to the National Cancer Institute.
This is where AI can help. Thanks to recent breakthroughs in computing, more hospitals are warming to the idea of using AI to spot what doctors might otherwise miss. In December, Intel and Penn Medicine announced they had helped researchers boost cancerous brain tumour detection by 33%.
Das, who is based in Kerala, India, explained how her team first turned to GPU-based options to boost their deep-learning models. There was just one problem – their GPU-based systems would often fail as the amount of compute required to process large images far exceeded the systems’ design limits, leading to freezes and frustrating restarts.
“Our models were computationally demanding, and our attempts to train the model using GPUs failed,” Nair wrote. “It took us several days to execute and we found it difficult to get the training done for higher-resolution images. That led us in search of a better computing facility.”
In 2022, Nair discovered the Intel opportunity when he brought up the problems he was facing with a Dell representative he met for a different research project. Dell introduced Nair to the Intel India team.
Months later, they deployed four servers operating as a single compute cluster without any deep learning accelerators. The servers and storage were connected using a high-speed Ethernet network.
On the software side, the joint team turned to Intel Optimization for TensorFlow, a software suite that boosts the performance of TensorFlow by taking advantage of acceleration features in Intel CPUs.
“I shared our problem with the Intel team and was extremely happy that they immediately understood the importance of this work,” Nair said. “They gave us the opportunity to use this distributed architecture.”
The results were exactly what the researchers were hoping for, with a bonus – their model doesn’t just flag cancerous cells, but also distinguishes different grades of cancer.
And it’s accurate. It achieved an accuracy of 98 per cent, about ten percentage points better than other models.
In time, as the team trains their model on more datasets, researchers expect that accuracy rate to inch upward.
“The Intel architecture was amazing,” said Nair. “We were able to complete the training in a few hours. Because the servers had 192Gbyte of memory, more than the 40Gbyte or 80Gbyte available on graphics cards, we were able to use high-resolution images and fit the whole model in memory. Intel also helped us to improve the model, and shared optimisations with us to get it working. That’s the reason why we were able to succeed.”
Das said the results were even more impressive given the accuracy figures were achieved with much less training data than other models needed, shortening turnaround times tremendously.
“We were really happy with the results,” she said. “It’s remarkable that we were able to achieve 98 per cent accuracy with only 20 per cent of the data annotated. That’s really exciting.”
Next up for the technology is waiting as the team secures patents and lands commercial partners. Some scans might involve consulting multiple pathologists, each lending his or her own conclusions based on their different experiences.
There’s also the subject of accuracy. While 98 per cent accuracy rates might seem high, it’s not yet precise enough for real-world applications.
Now that both Das and Nair have proven their model detects breast cancer cells reliably, they’re looking at applying a similar approach to cerebral aneurysms and classifying polyps from endoscopies.
“We’re also planning to expand this model to detect multiorgan cancer,” Das said, adding that she’s now working on extending it to analyse lymph node images, because breast cancer often spreads to the adjacent lymph nodes under the arm.